Big Data and Artificial Intelligence: How They Power One Another

26 Mar 2024
Igor Kelly
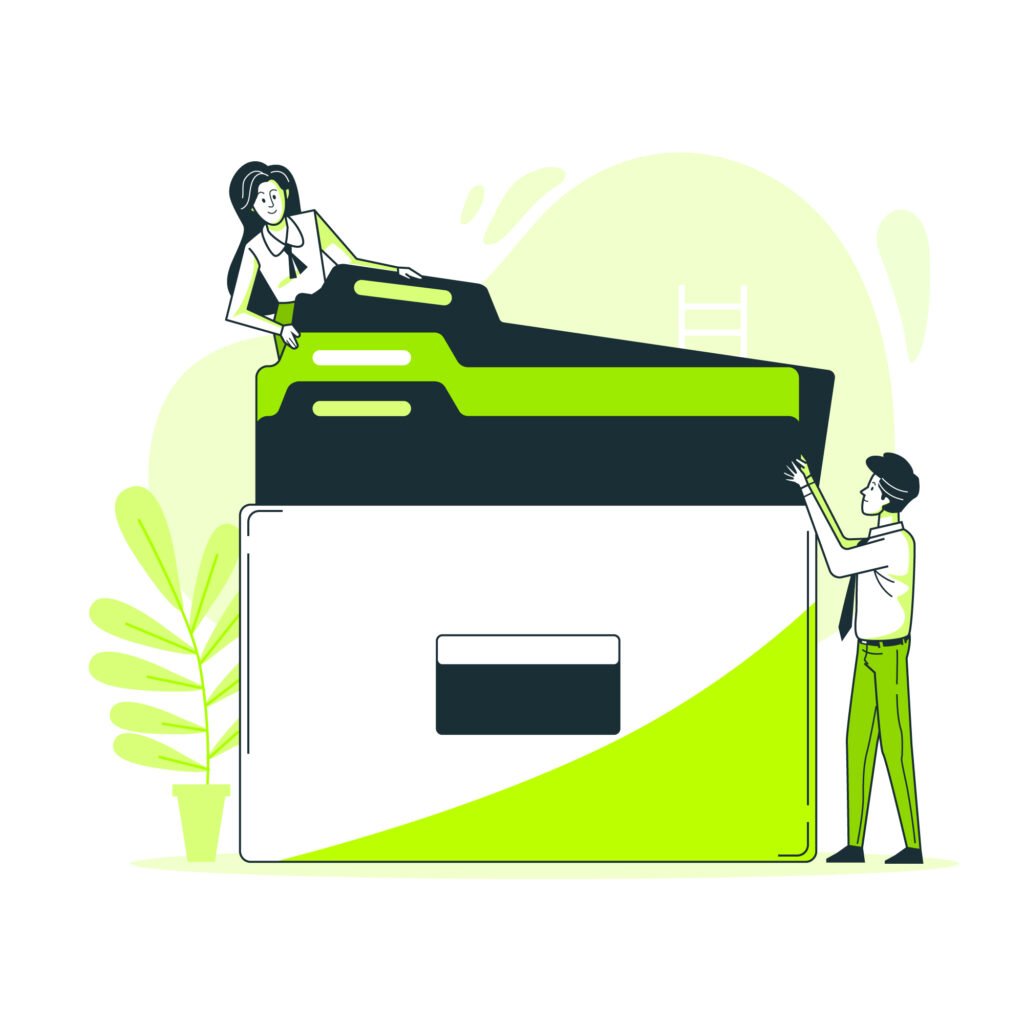
According to Statista, the volume of data produced annually worldwide will increase tenfold to 180 zettabytes by 2025. Not surprisingly, as our lives become increasingly interconnected, new data points are created for storage in many places. How can such big data create value and solve problems in our areas of interest and expertise?
In this article, we will explore some of the most inspiring and impressive examples of big data and AI in action, covering various sectors. We will also explore whether big data vs. artificial intelligence can be compared and how their synergistic integration drives the growth of modern companies.
Understanding Big Data and Artificial Intelligence
Big data combines unstructured, semi-structured, or structured data collected by organizations. This data can be evaluated to gain insights and used in projects for machine learning, predictive modeling, and other advanced analytics applications. Dealing with large data sets containing various data types comes from various sources listed below.
Big data source | Description |
Customer data | Data collected using CRM systems, including customer profiles, sales records, and customer interactions. |
Financial transactions | Data from banking systems, credit card transactions, stock markets, and other financial platforms. |
Healthcare data | Data from electronic health records (EHR), medical imaging, wearable health devices, clinical trials, and patient monitoring systems. |
Social media platforms | Data generated by social media platforms such as Facebook, Twitter, Instagram, and LinkedIn, including posts, comments, likes, shares, and user profiles. |
Web and mobile applications | Data generated by users when they interact with websites, mobile applications, and online services, including clicks, page views, and user behavior. |
Sensor networks | Data collected by environmental sensors, industrial machines, traffic monitoring systems, and other wireless sensor networks. |
Internet of Things (IoT) devices | Data collected from various IoT devices such as smart sensors, smart devices, wearable devices, and connected vehicles. |
E-commerce transactions | Data generated by online retail platforms, including customer orders, product details, payment information, and customer reviews. |
Big data is formally characterized by the five Vs:
- Volume describes the massive scope and size of data sets containing terabytes, petabytes, or exabytes of data.
- Velocity refers to the fast pace at which large amounts of new data are created.
- Variety describes the wide range of data types and formats that are produced.
- Veracity refers to how trustworthy and accurate the data is within a very large dataset.
- Value describes the ability of data to be transformed into actionable insights.
These characteristics have quickly overwhelmed the capabilities of traditional analytics. This is where AI comes into play.
Comparing big data vs. AI can be challenging because they are not mutually exclusive but rather complementary components of modern data-driven technologies. Here’s how they complement each other:
- Big data and AI are interdependent and often go hand in hand. Big data refers to the large volumes of data generated from various sources, while AI encompasses the algorithms and pattern recognition technologies used to analyze and derive insights from this data. Without big data, AI lacks the necessary input to function effectively, and without AI, big data has largely untapped potential.
- Big data and AI have a different focus. Big data primarily focuses on the management, storage, and processing of large datasets, whereas AI emphasizes the development of algorithms and models that can learn from data to perform specific tasks or make predictions. While big data deals with the infrastructure and tools needed to handle data at scale, AI is concerned with the cognitive computing and decision-making capabilities derived from that data.
- Big data and AI can be applied diversely. Big data and AI find applications across various industries and domains, from healthcare and finance to marketing and manufacturing. Their functionalities overlap in areas such as predictive analytics but also serve distinct purposes. For instance, big data might be used for data warehousing and analysis, while AI might be employed for natural language processing or computer vision tasks.
- Evolutionary nature of both concepts. Big data and AI are rapidly evolving fields, with new technologies, techniques, and methodologies emerging regularly. Attempting to compare them in static terms might overlook the dynamic nature of their development and their potential for convergence in the future.
While comparing big data vs. AI might provide some insights into their individual characteristics, recognizing their interdependence and understanding how they complement each other is essential for leveraging their combined potential effectively in solving real-world problems and driving innovation.
Big data development services design integrated data solutions and utilize complementary technologies for the following purposes:
- Data interpretation, processing, and analysis: AI techniques like machine learning analyze vast datasets to identify patterns and trends humans may miss.
- Predictive analytics: AI models and machine learning algorithms use historical data to make accurate predictions, aiding decision-making in finance, healthcare, marketing, and manufacturing.
- Personalization and recommendations: AI-powered recommendation systems, seen in platforms like Amazon and Netflix, leverage big data to provide personalized suggestions to users.
- Data quality improvement: AI tools automatically identify and rectify errors in big data, enhancing its quality and reliability.
- Real-time insights: AI enables real-time stream data analysis, crucial for industries like finance, cybersecurity, and IoT, facilitating predictive maintenance and immediate action based on insights.
AI-Driven Analytics: Transformative Applications
One of the best ways to learn more about big data and AI and their symbiotic relationship is to look at the success stories and case studies of leading companies and professionals who have used AI to solve real-world problems and create value. Here are some of the cases we will discuss:
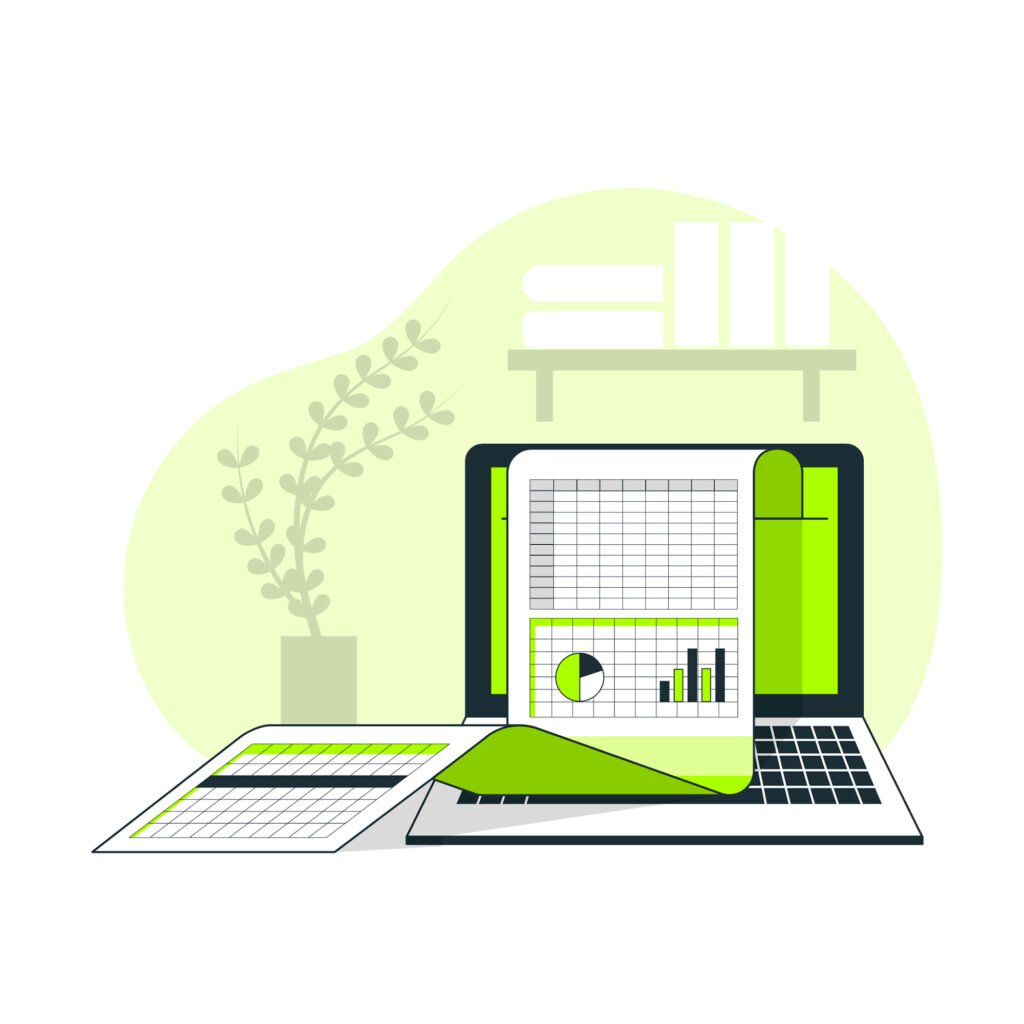
1. IBM Watson Health
IBM Watson is an AI-driven platform which can understand languages, analyze complex structured and unstructured data, and provide helpful insights. Watson Health is a branch of IBM Watson focused on the application of AI in healthcare and life sciences, to improve health outcomes, reduce costs, and promote research and innovation. Watson Health use cases include:
- Watson for Oncology: This cognitive computing system helps oncologists deliver personalized, evidence-based cancer treatment. Watson for Oncology leverages advanced analytics to examine a patient’s medical history, genetic information, and relevant clinical studies, offering personalized treatment recommendations and identifying suitable clinical trial opportunities tailored to each individual case.
- Watson for Drug Discovery: This cloud-based solution helps researchers discover new drugs and therapies by analyzing millions of scientific papers, patents, clinical trials, and other data sources. Watson for Drug Discovery harnesses big data and AI capabilities to uncover new drug targets, biomarkers, and mechanisms of action, while also forecasting the effectiveness and safety profiles of prospective compounds.
- Watson for Genomics: A service that integrates genomic sequencing data with the cognitive capabilities of Watson to deliver comprehensive and actionable insights for precision medicine. Watson for Genomics can identify relevant mutations, variants, and pathways and match them to the most appropriate drugs and clinical trials.
2. Google DeepMind
Google DeepMind is a research organization whose goal is to create artificial general intelligence (AGI), which is the ability of an AI system to perform any intellectual task that a human can. DeepMind is known for developing some of the most advanced and groundbreaking AI systems, such as:
- AlphaGo: This computer program can play the ancient and complex board game Go, considered one of the most demanding games for AI. AlphaGo made history in 2016 when it defeated Lee Sedol, one of the world’s best Go players, in a five-game match. AlphaGo later evolved into AlphaGo Zero, which learned to play Go from scratch without human knowledge, and AlphaZero, which also mastered chess and shogi.
- DeepMind Health: This division of DeepMind applies AI to healthcare and biomedical research intending to improve the quality and accessibility of care. DeepMind Health has worked with several hospitals and organizations to develop and deploy AI solutions such as:
- Streams: This mobile app helps doctors monitor patients with acute kidney injury, sepsis, and other conditions. Streams can inform doctors about any changes in the patient’s condition and provide them with relevant information and guidance.
- Verily: This joint venture between DeepMind and Google’s parent company, Alphabet, focused on using AI and other technologies to improve health and well-being. Verily has launched several projects and initiatives, including two initiatives.
One is a longitudinal study to collect and analyze comprehensive health data from thousands of participants and use AI to understand the factors influencing health and disease. Another is a virtual diabetes clinic that uses AI and connected devices to provide personalized coaching, support, and treatment for people with type 2 diabetes.
3. Netflix
Netflix is a global leader in online streaming and entertainment, with over 200 million subscribers and an extensive library of original and licensed content. Netflix uses big data and machine learning to improve its products and services, such as:
- Recommendation system: This system analyzes the user’s preferences, behavior and feedback and suggests the most relevant and engaging content to each user. Netflix’s recommendation system is responsible for more than 80% of the content users watch on the platform, helping Netflix retain and satisfy its customers.
- Content creation: This process uses big data and machine learning to inform and optimize the production and distribution of Netflix’s original content. Netflix uses AI to analyze market trends, audience demand, and content performance. Data-driven decision-making refines what type of content to create, how much to invest, and how to market and deliver it. Netflix also uses AI to assist with various aspects of content creation, such as script writing, casting, editing, and dubbing.
In addition to business intelligence across big market players, there is a whole range of other application areas for big data analytics. The analysis of large amounts of data can be used, for example, in the fight against crime and the insurance industry, for risk assessment and adjustment of insurance premiums. Other possible application examples include processing weather data, evaluating movement profiles, or analyzing web statistics. However, all this diversity faces challenges, which we will discuss below.
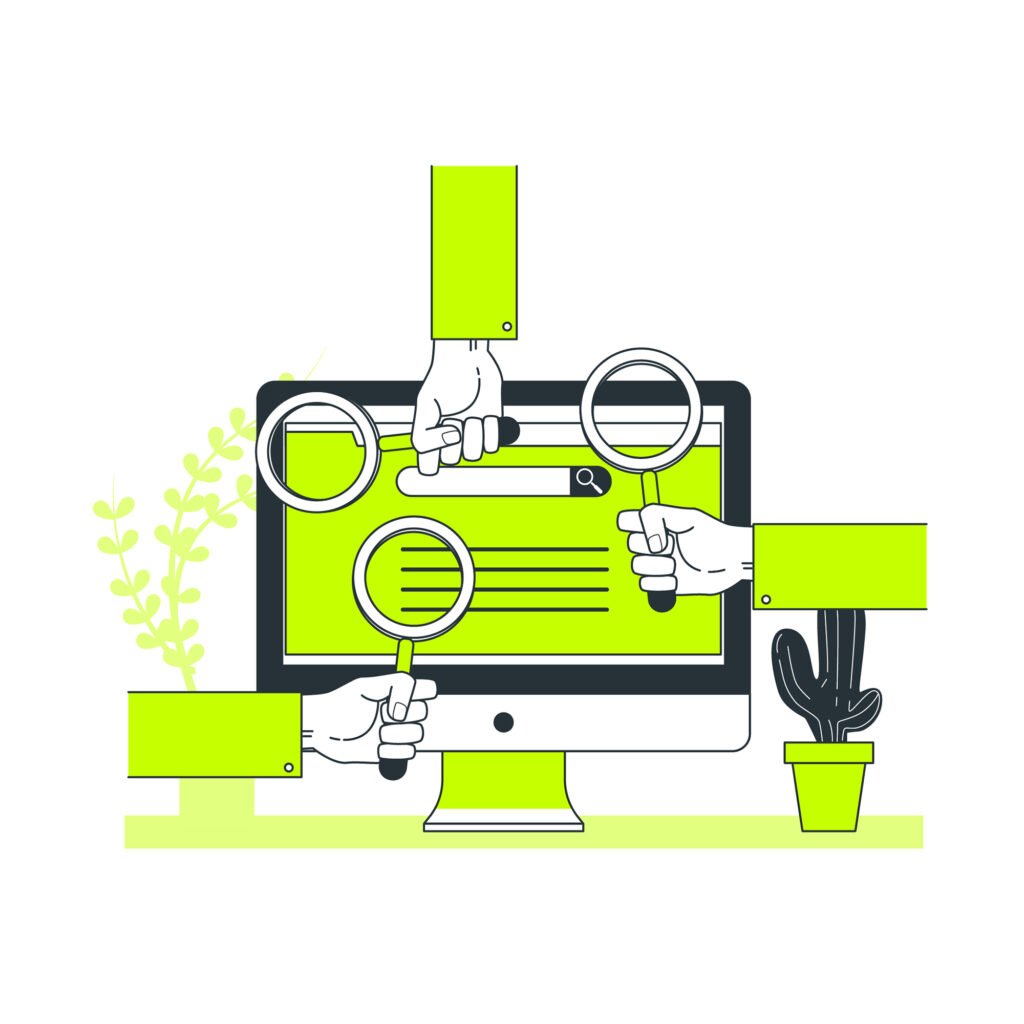
Challenges and Limitations
Just as it is difficult to find a specific object in a cluttered room, such large databases can ironically make it difficult to find useful and relevant data. Let’s look at a few frequent challenges to adopting big data and AI.
- Data analysis: The more data available, the more accurate the conclusions tend to be. However, drawing conclusions from huge data sets can be difficult because traditional software struggles to process such large quantities (and big data far exceeds the analytical capacity of non-technological humans).
- Retrieving data: Retrieving data can be expensive, especially if the data is stored in the cloud. Object storage is low maintenance and virtually unlimited, making it ideal for large amounts of data. However, object storage providers often charge egress fees to access the stored data.
- Ensuring data accuracy: Inaccurate or unreliable data causes predictive models and machine learning algorithms trained on that data to produce incorrect results. However, verifying the accuracy of large and rapidly growing amounts of data in real time is difficult.
- Data protection and legal concerns: Big data collections may contain data that has been classified as personal data under legal frameworks such as the GDPR. Even if a data set does not currently contain such data, a new legal framework may expand the definition of personal information so that the stored data falls under the new rules. A company may not be aware that its records contain such data but may face fines and penalties if this data is accessed or misused. Additionally, if a database contains personal data, the owner of the database has greater liability in the event of a data breach.
AI software development services offer valuable support to companies aiming to implement new technologies by assisting in overcoming the challenges above. Skilled outsourced teams enable companies to justify their investments in AI and big data initiatives by optimizing data processing, enhancing predictive modeling accuracy, ensuring compliance with evolving legal frameworks, mitigating risks, and maximizing the benefits of adopting advanced technologies.
Future Trends and Outlook
The big goal of big data and AI development is an AI with a human-like mind that can successfully master various tasks and even outperform humans. According to our current understanding, we are at least several decades away from such an artificial general intelligence (AGI).
According to a study conducted in 2019, 45 percent of the AI experts surveyed estimated the time for the development of an AGI to be 2060 or earlier, 34 percent estimated later, while 21 percent of those surveyed considered the existence of an AGI to be completely impossible.
How will big data analytics and artificial intelligence develop in 2024? Let’s look at a few predictions.
- Continued high pace of development: The pace of innovation will continue in 2024 The market will be flooded with new applications. Technological development in generative AI will progress dynamically; advanced technologies, like Large Language Models (LLM), are already integrated into numerous products and services.
- Neural networks: Convolutional Neural Networks (CNNs) are a type of deep learning algorithm primarily used for visual recognition and analysis tasks, such as image classification, object detection, and image segmentation. They are designed to automatically and adaptively learn spatial hierarchies of features from input data. These networks are modeled on the human brain’s visual cortex structure, promising breakthroughs in autonomous driving, medical imaging diagnostics, and surveillance systems.
- Ethical AI development: As the initial excitement around AI fades, companies increasingly prioritize ethical considerations in data usage. Scientists predict that the conscientious and secure application of innovative AI models will become paramount, involving IT departments, management, and legal teams. This trend signifies a shift towards ethical AI development, where responsible data stewardship and privacy protection are integral to AI innovation and adoption.
Conclusion
The collaborative intelligence of big data and AI is transforming the business world and creating new opportunities for growth, innovation, and competitive advantage. However, AI also presents major challenges for companies that must weigh the benefits and risks of using this powerful technology. In their vision of “The data-driven enterprise of 2025,” McKinsey & Company outlines seven defining traits:
- Integration of data into decision-making processes.
- Real-time data processing capabilities.
- Utilization of adaptable data storage solutions for immediate access.
- Treatment of data assets as valuable products.
- Expectation for Chief Data Officers (CDOs) to drive value creation.
- Adoption of data-sharing platforms as standard practice.
- Elevated focus on effective data management strategies.
Would you like to explore the potential of AI-supported data analytics to future-proof your company?Contact us for non-binding advice from experienced experts.