5 Examples of Predictive Analytics in Martech

2 Apr 2024
Igor Kelly
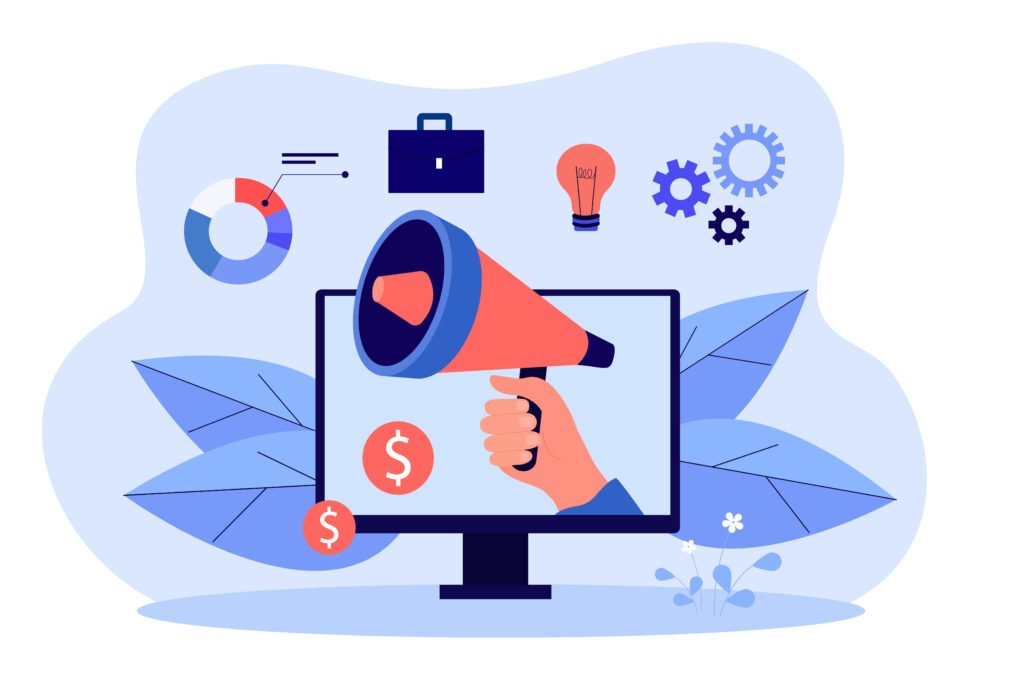
Being fast is no longer enough. Customers expect their wishes to be read from their lips right from the very first contact. In addition, as organizations accumulate larger and broader data pools, they seek more advanced data mining opportunities to gain predictive insights. And modern data analysis methods, such as predictive analytics, make exactly that possible.
Predictive analytics in marketing enables forecasting customer behavior, optimizing campaigns, personalizing content, and enhancing decision-making for marketers, and therefore it belongs in every AI-driven Martech stack. In this article, we delve into the world of predictive analytics and examine practical use cases on how companies bring their marketing strategies to the next level.
Predictive Analytics Overview
A predictive analytics process or approach consists of a series of steps that build on each other. The most commonly used models in predictive analytics are classification and regression algorithms.
- Classification algorithms sort (or classify) data by category. Is this person female or male? Is this email spam or not?
- Regression algorithms are used to predict a numerical result. Will the price go up or down? How many customers can a new business expect?
The entire process of creating questions is iterative, meaning you approach the desired result in several iterations; the steps can be repeated. Here’s a general algorithm of the predictive modeling work:
- Setting the objectives: At the initial step, the goals of the analysis for the company and the industry are determined. The data sources and their availability are also checked here.
- Data collection: The unifying data collection from different data sources takes place second.
- Checking and processing the data: The most important thing about data is its quality and delivery reliability. Therefore, the data is checked, cleaned, and available for upcoming analyses after collection.
- Building predictive models: Accurate predictive models are built. Future forecasts can either refer to points in time and values (such as sales forecasts for a defined point in time) or classifications (such as a risk analysis).
- Model testing and adjustment: The models are «fed» with the data and tested, and any corrections are made.
- Provision for integration into company processes: Now, you can use the insights for decisions in the company to analyze customer behavior more attentively.
Personalized Customer Recommendations
Personalization in marketing means that communication is tailored to the individual target customer. It is no longer just individualized targeting but sometimes also advertising messages, content, offers, or even prices. It’s no longer just a matter of personal salutation in the newsletter. In a recent study, 80% of people said they prefer doing business with companies that offer personalized experiences. Additionally, an impressive 90% mentioned that they find personalization desirable.
What does personalization bring? From the user’s perspective, personalization means convenience: they have to filter out less irrelevant information to reach their goal. In turn, this results in a higher conversion rate for the marketer.
Personalization in marketing usually goes through two or three phases:
- Identification of the user
- If necessary, assignation of the user to a group (categorization)
- Providing content by the rules (generating content «on-demand» if necessary)
Here are the most widely used examples of Martech platforms using predictive algorithms to optimize content delivery:
- Adobe Experience Cloud employs predictive algorithms via Adobe Target to optimize content delivery, analyzing customer data to predict content variations with the highest engagement and conversion rates.
- Salesforce Marketing Cloud utilizes predictive analytics, including Einstein Content Selection, to automatically deliver relevant content based on individual customer interactions and preferences, enhancing engagement and conversion.
- HubSpot Marketing Hub employs predictive algorithms in its Content Strategy feature to recommend content topics, formats, and distribution channels based on past campaign data and audience interactions, driving targeted content creation and ROI optimization.
- Google Marketing Platform utilizes predictive algorithms, such as Smart Bidding and Responsive Search Ads, to analyze user behavior and optimize content delivery across digital channels, ensuring effective campaigns with minimal manual intervention.
As privacy concerns mount and browser updates restrict third-party cookies, predictive analytics and AI will become essential for hyperpersonalized marketing, relying on thorough analysis of both first- and third-party data to anticipate customer behavior and assess customer lifetime value. Martech development keeps up with the times and enables automatic content optimization by analyzing user engagement metrics and predicting future trends, ensuring content remains relevant and impactful.
The success of many market giants has been built precisely on a well-crafted approach to personalizing recommendations for customers. Let’s examine the highlighted below.
- Amazon: Amazon’s personalized recommendation engine analyzes user data to suggest tailored products, driving increased sales and satisfaction. These recommendations contribute significantly to Amazon’s revenue, which is estimated at 35% of total sales. Through continuous optimization, Amazon fosters long-term customer loyalty.
- Netflix: Netflix’s recommendation system analyzes viewing habits to receive customer insights and suggest content, enhancing user experience, engagement, and retention. Studies show personalized recommendations are crucial in keeping subscribers engaged, driving significant viewing hours.
- Spotify: Spotify’s personalized playlists, like Discover Weekly, analyze user data to introduce tailored music experiences, fostering deeper user connections. Research indicates personalized playlists significantly impact user engagement and retention, making Spotify a leader in personalized music streaming.
Predictive analytics marketing improves your value proposition, helps you compete in an increasingly tight market, ensures stronger customer relationships, and increases the efficiency of your processes.
Customer Churn Prediction
It is often not easy to see to what extent customer losses and churn are homemade or the result of external trends. So-called churn prediction software helps to correctly classify such fluctuation processes and take appropriate countermeasures.
Let’s examine a few industries where predictive analytics helps identify customers likely to churn.
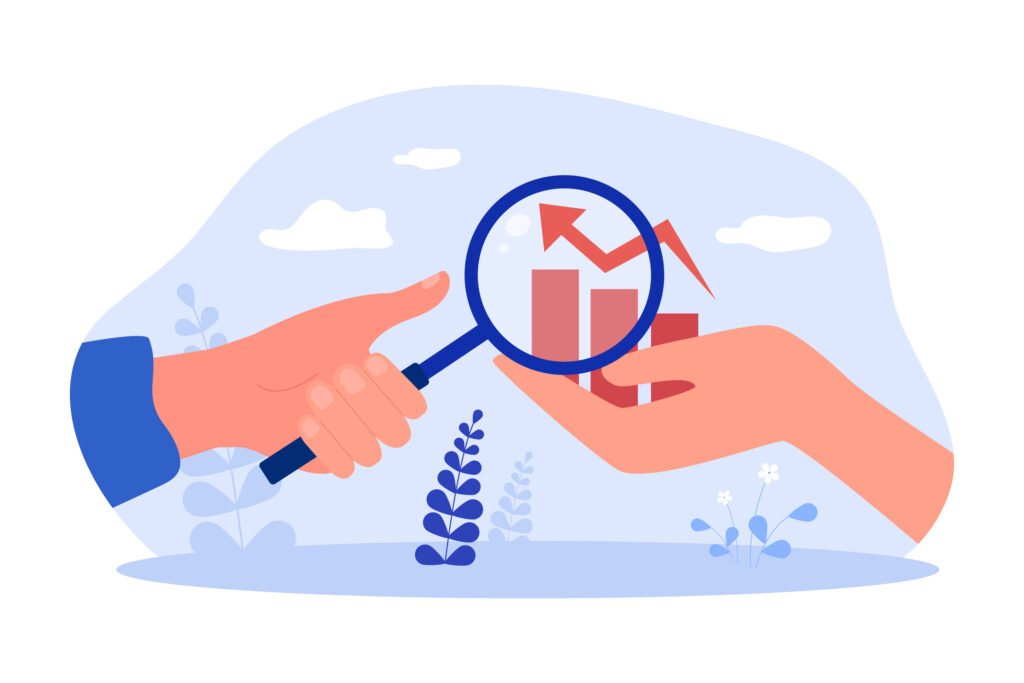
Proactive measures in the telecommunications industry
In the telecommunications sector, customer churn poses a significant challenge due to fierce competition and evolving consumer preferences.
Churn prediction software analyzes:
- Customer usage patterns
- Service interactions
- Billing history
to identify signals indicative of potential churn, such as:
- Declining usage
- Late payments
- Customer complaints
By leveraging predictive analytics marketing, telecom companies can intervene before customers switch providers by offering targeted retention campaigns. This could be a service upgrade to a premium plan with a larger data allocation at a discounted rate. The offer is often communicated through targeted emails or SMS messages, highlighting the benefits of the upgraded plan, such as unlimited data usage or bonus features like free access to streaming services.
Maintaining customer loyalty in the E-commerce industry
In the e-commerce sector, retaining customers is critical for sustaining growth and profitability. Churn prediction software analyzes:
- Customer purchase history
- Browsing сustomer behavior
- Engagement metrics
to identify customers showing signs of disengagement, such as:
- Decreased purchasing frequency
- Abandoned carts
By applying predictive analytics, e-commerce platforms can implement targeted re-engagement strategies, such as personalized email offers, dynamic discounts, or loyalty incentives, to prevent customers from defecting to competitors. For example, an online retailer used predictive analytics software to identify dormant customers and sent targeted emails with exclusive discounts or product recommendations based on past purchases, significantly increasing reactivation rates.
Engagement strategies in the financial services industry
Customer churn can have profound implications for revenue generation and market share in the financial services sector. Churn prediction software analyzes:
- Customer transaction data
- Account activity
- Service interactions
to identify customers exhibiting behaviors indicative of potential attrition, such as:
- Reduced account balances
- Decreased transaction frequency
- Negative sentiment in customer feedback
By leveraging predictive analytics, banks and financial institutions can implement proactive retention strategies, such as personalized financial advisory services, loyalty rewards programs, or targeted promotional offers, to mitigate churn risk and enhance customer satisfaction. For instance, a leading bank utilized churn prediction software to identify customers likely to switch to competitors and proactively reached out with personalized offers, significantly reducing churn rates and increasing customer retention.
In each scenario, customer churn prediction software empowered businesses to accurately identify customers at risk of churn and take timely countermeasures to retain them. These activities usually include the following:
- Define metrics: Establish key metrics like customer retention rate, churn rate, lifetime value, and satisfaction scores to measure churn reduction efforts. For instance, track changes in churn rate post-implementation of a personalized email campaign for at-risk customers.
- Collect and analyze feedback: Gather feedback through surveys, interviews, or online reviews to understand customer satisfaction and churn drivers. Define areas needing improvement, such as enhancing customer service processes.
- A/B testing: Implement A/B testing to assess the effectiveness of different strategies. Experiment with variations to optimize initiatives based on real-time data, enabling quick adjustments and maximizing efficiency.
Overall, timely measures drive revenue growth, enhance customer satisfaction, and maintain competitive advantage in dynamic market environments.
Lead Scoring and Prioritization
Quickly identifying which leads are ready to buy is crucial to improve probability of closing the deal. Lead scoring is a way to rank prospects based on their importance to a company. Traditionally, this process is done manually by sales and marketing teams, who decide on criteria like ebook downloads or webinar attendance to assign points indicating purchase readiness.
For example, a lead might earn points for actions like downloading an ebook or attending a webinar, with more points assigned for behaviors deemed more indicative of a readiness to buy. You can compare how the process changes with process automation, enabled by predictive analytics software:
Criteria | Traditional Lead Scoring | Lead Scoring with Predictive Analytics Software |
Data Collection | Manual process based on past experiences and intuition | Automated real-time analytics of vast data sets, including customer interactions, demographics, etc. |
Scoring Method | Points assigned for predefined behaviors | Machine learning algorithms analyze data to assign scores based on predictive indicators |
Flexibility and Accuracy | Limited adaptability and reliance on subjective judgment | Highly adaptable with dynamic scoring models that evolve based on real-time insights |
Modern AI-based predictive lead scoring has three significant advantages over traditional lead and customer scoring systems:
- Lead qualification improvement: Predictive analytics prioritizes leads based on conversion likelihood by analyzing past data for patterns. For instance, a software company can identify leads with prior engagement in similar products or specific feature interests, aiding sales teams in focusing efforts on high-potential leads. This enhances lead qualification, boosting conversion rates through targeted engagement.
- Enhanced personalization: Predictive analytics enables personalized interactions with leads, fostering better engagement and relationship building. Predictive models offer insights into lead preferences by analyzing demographics, browsing behavior, and purchase history. For example, an E-commerce company tailors marketing messages based on a lead’s preferred product categories, which enhances customer satisfaction and conversion rates.
- Identifying upselling/cross-selling opportunities: Predictive analytics uncovers upselling and cross-selling opportunities through customer behavior analysis. By understanding preferences, predictive models recommend relevant products or services. For instance, an online streaming platform analyzes viewing history to suggest similar content. Leveraging predictive analytics in lead scoring boosts sales and enhances personalized customer experiences.
The accuracy of predictive analytics in marketing relies heavily on data quality, which can compromise outdated information, duplicates, or incorrect entries. These issues distort predictive models, potentially leading sales teams to pursue low-quality leads or miss high-potential ones. To address this, industries that use predictive analytics need robust data governance policies, including clear standards for data entry and tailored predictive analytics software development for identifying and rectifying inconsistencies.
Dynamic Pricing Strategies
Using fixed prices and long-term contracts has long been a proven strategy in B2B wholesale to ensure stability and predictability. However, in the age of dynamic pricing, these rigid pricing structures could be fatal for businesses. With the help of predictive analytics marketing, companies can determine the optimal final price for each customer and implement flexible pricing strategies that adapt to current market conditions.
Predictive analytics calculates dynamic pricing by analyzing various factors such as demand, competitor pricing, customer behavior, and market trends in real-time to determine the optimal price for products or services, maximizing revenue and profitability. Here are the benefits noted by industries that use predictive analytics for refining pricing strategies:
- Revenue maximization: Dynamic pricing strategies enable businesses to optimize revenue by strategically adjusting prices in real time, ensuring they capture the highest possible value from each transaction. For example, a hotel chain might implement dynamic pricing during peak seasons to adjust room rates based on demand, maximizing revenue potential.
- Better supply-demand balancing: Leveraging dynamic pricing allows businesses to manage supply and demand fluctuations effectively by adapting prices to match changing market conditions. For instance, a ride-sharing company may increase prices during times of high demand, such as rush hours or adverse weather conditions, to balance driver availability and passenger demand.
- Faster market adaptability: Dynamic pricing provides businesses with a competitive edge by enabling swift responses to market changes. By continuously monitoring and adjusting prices, companies can stay ahead of competitors and capitalize on emerging opportunities. For example, an e-commerce retailer can dynamically adjust prices based on competitor pricing changes, ensuring they remain competitive.
- Personalized pricing: Personalized pricing empowers businesses to effectively segment their customer base, tailoring pricing incentives to individual preferences and behaviors. Companies can tailor prices to specific customer segments by analyzing customer behavior and purchase history, enhancing customer satisfaction and loyalty. For instance, a subscription-based streaming service might offer personalized pricing plans based on individual viewing preferences and usage patterns, thus increasing the likelihood of the subscription renewal.
Also, the use of dynamic pricing in combination with churn prediction discussed above has proven to be a powerful combination. While churn prediction determines when action needs to be taken, dynamic pricing defines the action.
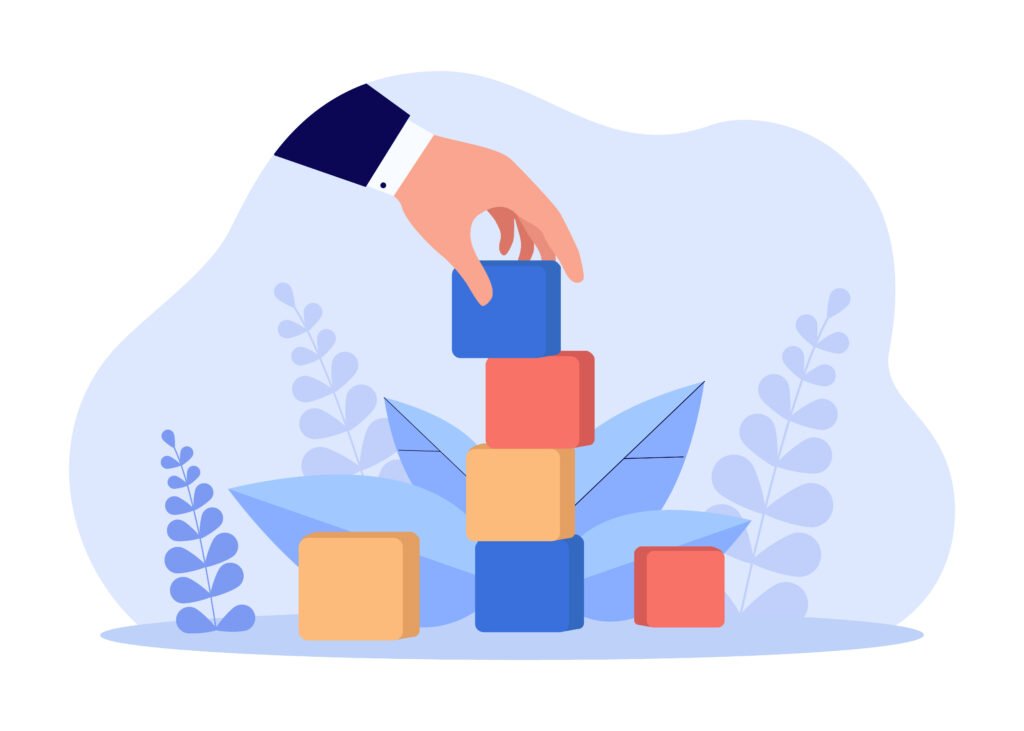
Integration of Predictive Analytics in Martech Ecosystem
Over the years, ever larger amounts of data accumulate in a company. This is not least because digital technologies constantly evolve and offer the opportunity to obtain new data sets. All of this information could not be evaluated manually by humans. That’s why data science uses predictive analytics to benefit from the data sets.
Where to start when integrating predictive analytics into the existing marketing technology stack? At Lightpoint, we adhere to the following steps when implementing predictive analytics tools:
1. Assessment and planning:
- Conduct a comprehensive assessment of the customer’s existing marketing technology infrastructure, including data sources, storage systems, analytics tools, and processes.
- Identify key stakeholders and gather requirements to understand the customer’s needs, goals, and challenges.
- Develop a detailed implementation plan outlining timelines, resource requirements, and milestones.
2. Data integration and preparation:
- Integrate data from various sources such as CRM systems, website analytics, social media platforms, and third-party data providers.
- Cleanse and preprocess the data to ensure accuracy, consistency, and completeness.
- Establish data pipelines and workflows to automate data collection, transformation, and loading processes.
3. Model development and testing:
- Develop predictive models tailored to the customer’s objectives, such as customer segmentation, churn prediction, lead scoring, or campaign optimization.
- Train and fine-tune the models using historical data, leveraging machine learning, statistical analysis, and data mining techniques.
- Validate the models using cross-validation techniques and assess their performance against predefined metrics and benchmarks.
4. Integration and deployment:
- Integrate the predictive models into the customer’s existing marketing technology stack, ensuring compatibility and seamless interoperability with other tools and systems.
- Deploy the models into production environments, whether on-premises or in the cloud, and configure them to generate real-time predictions and insights.
- Conduct thorough testing and validation to ensure the reliability, scalability, and performance of the deployed models in live production environments.
5. Training and knowledge transfer:
- Provide training sessions and workshops to educate the customer’s team on effectively interpreting and leveraging predictive analytics insights.
- Document best practices, guidelines, and troubleshooting procedures to empower the customer’s team to independently maintain and optimize the predictive analytics systems.
After the implementation, we continuously monitor the performance and accuracy of the predictive models, identifying potential drift retraining the models as needed to ensure their relevance and effectiveness.
Conclusion
No matter how much data you gather and analyze, predicting the future with 100% accuracy is still impossible. However, marketers can forecast probable outcomes with high precision using predictive analytics marketing. Predictive data modeling helps to use data gathered from in-house and third-party sources to uncover patterns, outliers, and other key indicators to identify likely outcomes in various scenarios.
With these insights, marketing teams optimize their campaigns to meet behavior, event-based, or revenue goals in content optimization, campaign performance improvement, and many more ad hoc situations. By using predictive analytics tools, businesses can better meet the following KPIs:
- Reduce excess inventory holding costs
- Forecast customer lifetime value more accurately
- Anticipate demand fluctuations
- Get better return on ad spend (ROAS), and more
To target group-oriented customer care and ensure that resources are focused on the most promising contacts, seek advice from the Lightpoint expert today.