- Predictive Customer Analytics Vs Traditional One
- The Power of Anticipation: Benefits of Predictive Customer Analytics
- Algorithms Used In Predictive Models for Customer Behavior
- Real-World Impact: Case Studies in Predictive Customer Analytics
- Implementing Predictive Behavior Modeling: Challenges and Tips
- Ethical Considerations in Predictive Customer Analytics
- Future Trends: The Evolution of Predictive Customer Analytics
- Conclusion
How Predictive Customer Analytics Anticipates Needs and Preferences

30 Oct 2023
Igor Kelly
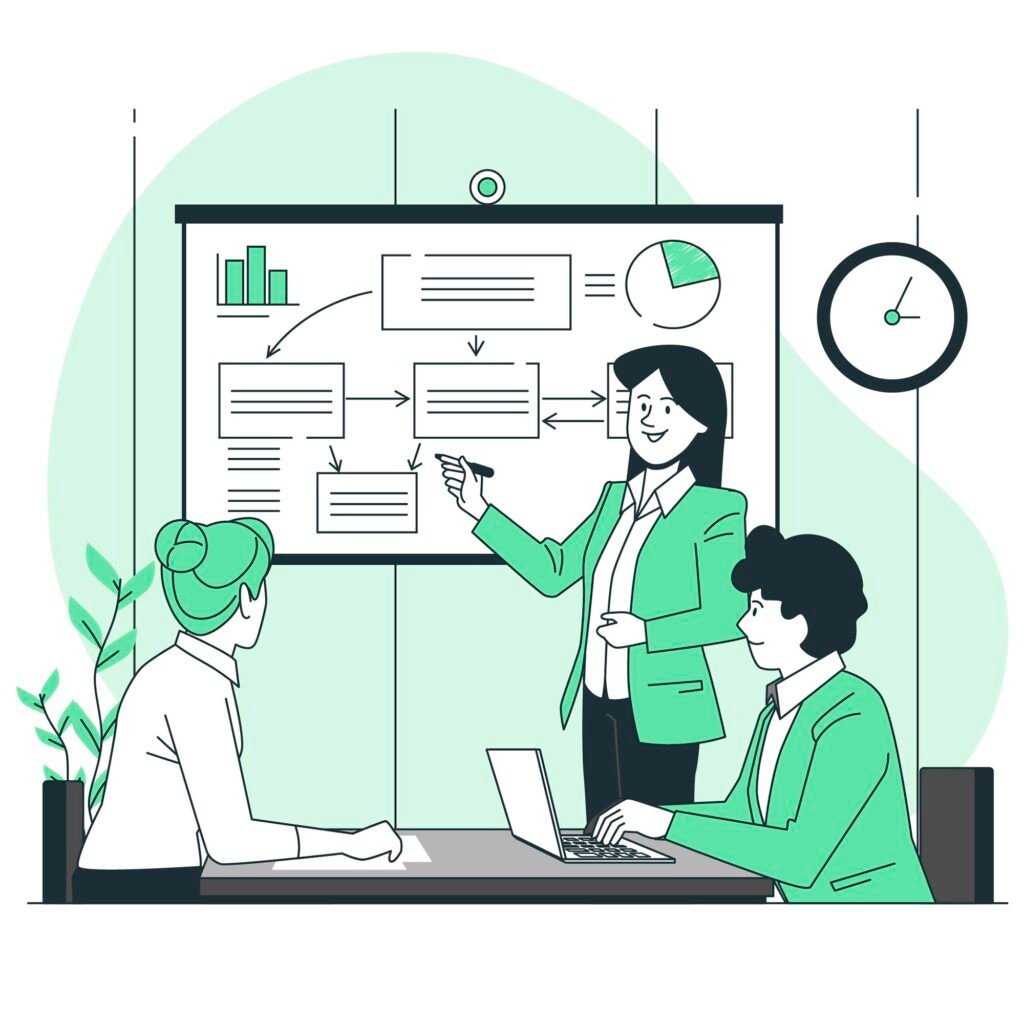
Think of predictive customer analytics for a business as a magical crystal ball that can predict what your customers might do next.In this article, we delve into the significance of predictive customer analytics for customer-centric approach. You will discover how marketing automation empowers businesses to decipher the intricate patterns of consumer preferences, predict future actions, and tailor personalized experiences accordingly.
Predictive Customer Analytics Vs Traditional One
Predictive analytics is data mining that uses statistical models and algorithms to predict future outcomes or behaviors. Companies use it to identify trends and predict changes in customer behavior or demand for products or services. By using predictive analytics in manufacturing, companies can improve their production processes, reduce downtime due to machine breakdowns or other problems, optimize inventory levels, and manage the supply chain more effectively.
Predictive analytics excels over traditional analytics in several ways:
- Proactive decision-making: Traditional analytics, focused on historical data, is more reactive and may not provide insights into emerging trends or potential issues. Predictive analytics enables businesses to take proactive actions based on future predictions.
- Optimized resource allocation: Predictive analytics helps allocate resources more efficiently. By forecasting future demands, companies can optimize inventory, staff, and budgets, reducing waste and improving cost-effectiveness. Traditional analytics may not offer such forward-looking resource planning.
- Competitive advantage: The ability to anticipate customer behavior, market intelligence, and emerging risks gives businesses a competitive edge. Predictive analytics allows companies to position themselves strategically in the market, create unique customer loyalty programs, and stay ahead of competitors, which traditional analytics alone may not achieve.
This forward-looking approach empowers businesses to make proactive decisions, optimize strategies, and respond to potential opportunities or challenges before they occur, enhancing their competitiveness and strategic advantage.
The Power of Anticipation: Benefits of Predictive Customer Analytics
The right use of data ensures greater efficiency, profitability, and unforgettable shopping experiences. Check out the proven benefits of using predictive analytics for business.
Data-driven insights
Predictive analytics leverages historical data and machine learning algorithms to provide data-driven insights into customer behavior, for example, recognizing which products or services are likely to resonate with specific customers, enabling targeted upsell and cross-sell campaigns.This enables businesses to make informed decisions based on evidence rather than assumptions.
Improved customer segmentation
Predictive analytics helps businesses segment their customer base more effectively. By identifying patterns and trends in customer data, businesses can target specific customer segments with personalized marketing campaigns, products, and services.
Enhanced customer retention
Understanding customer behavior through predictive analytics allows businesses to proactively identify customers at risk of churning. For example, if a customer starts showing signs of reduced engagement, such as fewer logins, decreased usage of a service, or fewer interactions with the company’s communication, the system can detect a drop in their activity level. This enables them to take preventive actions, such as personalized offers or outreach, to retain valuable customers.
Optimized marketing campaigns
Predictive intelligence can fine-tune marketing campaigns by predicting which channels, messaging, and timing will most likely resonate with individual customers. For instance, a clothing retailer can use past customer behavior to send targeted email promotions for winter collections at the ideal time, boosting engagement and sales.
Forecasting and planning
Businesses can use predictive analytics to forecast future customer behavior, such as sales trends and demand patterns. For instance, by analyzing historical data on past holiday sales, customer preferences, and market conditions, the system can predict the likely sales trajectory for specific product categories. This aids in inventory management, resource allocation, and long-term strategic planning.
These advantages demonstrate the value of predictive analytics in gaining deeper insights into customer behavior, enabling businesses to keep up with customer-centric marketing.
Algorithms Used In Predictive Models for Customer Behavior
The technical background of predictive analytics are specific models for customer behavior that utilize historical data and ML algorithms to forecast how customers are likely to act in the future.
These models help businesses understand and anticipate behavioral patterns and responses to marketing strategies, enabling data-driven decision-making and more effective customer engagement. Some common algorithms used include:
- Logistic regression is widely used for binary classification problems, such as predicting whether a customer will churn or not based on historical data analysis.
- Decision trees are used for classification tasks and are interpretable, making them valuable for understanding the factors influencing behavior.
- Random forest, an ensemble learning technique, builds multiple decision trees to improve prediction accuracy and reduce overfitting.
- Gradient Boosting Machines (GBM) algorithms like XGBoost and LightGBM are known for their predictive power and are often used for classification tasks in behavioral analytics.
- Neural networks, or deep learning models, such as artificial neural networks (ANNs), are used for complex tasks like image and text analysis in behavioral modeling when large datasets are available.
- Support Vector Machines (SVMs) are effective for classification and regression tasks, mainly when dealing with high-dimensional data.
- Hidden Markov models (HMMs) are used in sequential data modeling, making them valuable for tasks like predicting customer journey paths.
- Time series analysis techniques like ARIMA (AutoRegressive Integrated Moving Average) and LSTM (Long Short-Term Memory) recurrent neural networks are employed for behavioral modeling involving time-dependent data.
The choice of algorithm depends on factors such as the data’s nature, the problem’s complexity, the need for interpretability, and the available computational resources. Often, a combination of algorithms and ensemble methods is used to improve predictive performance in behavioral pattern recognition.
Real-World Impact: Case Studies in Predictive Customer Analytics
Understanding what is predictive behavior modeling is crucial for companies seeking to proactively address customer needs and optimize their strategies based on future data predictions. To illustrate how customer predictive analytics tools work in practice, we assembled three success stories from different industries.
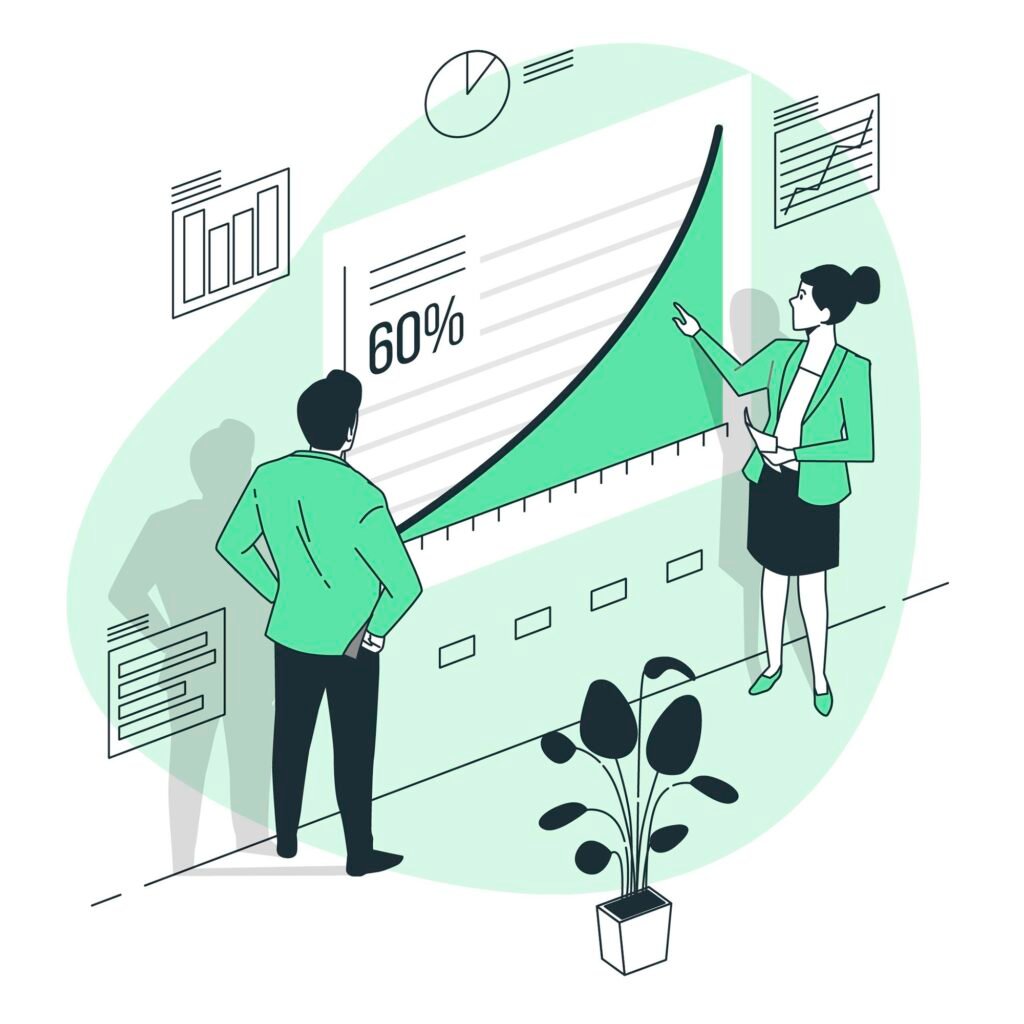
Case study №1: Retail industry
Challenge: A large retail chain was facing high customer churn rates. They had no clear understanding of why customers were leaving, and their traditional marketing efforts were ineffective in retaining them.
Solution: By adopting customer predictive analytics, the retailer analyzed historical purchase data, customer demographics, and behavior. The analytics solution revealed that certain customer segments were more likely to churn, often after specific trigger events, such as long periods of inactivity.
Armed with this insight, the retailer implemented personalized email campaigns and incentives targeting these at-risk segments, enticing them to return and purchase. They also optimized inventory management to reduce stockouts, improving the shopping experience. As a result, the retailer saw a 20% reduction in churn rates and a corresponding increase in revenue.
Case study №2: Telecom industry
Challenge: A telecommunications company faced fierce competition and a high rate of customer defection to competitors. They needed a way to retain existing customers and reduce customer acquisition costs.
Solution: The company adopted customer predictive analytics to analyze customer data, including call logs, usage patterns, and billing history. Predictive models were used to identify customers most likely to switch providers based on behavior, such as increasing complaints or frequent calls to the customer service department. With this insight, the telecom company proactively contacted these customers with tailored retention offers and discounts. Over time, the company reduced churn rates by 15% and increased customer lifetime value, leading to substantial cost savings.
Case study №3: Healthcare industry
Challenge: A healthcare insurance provider faced a growing issue of policyholders dropping their coverage. This not only led to revenue loss but also disrupted the continuity of care for many individuals.
Solution: The insurer analyzed historical claims data and member information using customer predictive analytics. They identified patterns indicating when policyholders were more likely to cancel their policies, often after significant life events or changes in healthcare needs. They also provided educational materials to help members better understand their policies. As a result, the insurer reduced policy cancellations by 18%, improved customer satisfaction, and increased the long-term value of their customer relationships.
These case studies highlight how predictive analytics can effectively supplement customer retention strategies across different industries. By leveraging data-driven customer insights, businesses can proactively engage with customers, tailor their marketing strategies, and ultimately enhance customer loyalty while achieving substantial financial benefits.
Implementing Predictive Behavior Modeling: Challenges and Tips
The cases above may sound inspiring, but there is much work to be done and many details to consider before implementing predictive behavior modeling. To ensure a smooth implementation process, businesses should bear in mind the following key points:
- Data quality and availability. Ensure that high-quality, relevant, and sufficient data is accessible for modeling, as the accuracy and effectiveness of predictions depend on the data quality.
- Clear objectives. Define clear business objectives and use cases for predictive modeling to ensure they align with strategic goals and address specific challenges or opportunities.
- Data privacy and compliance. Establish robust data privacy measures and ensure compliance with relevant regulations, such as GDPR or CCPA, to protect customer information and maintain trust.
- Expertise and resources. Have access to the necessary expertise, talent, and resources to develop, deploy, and maintain predictive models effectively, considering factors like data science skills and computational resources.
Various tools are available for predictive behavior modeling, catering to enhanced user experience, business needs, and technical expertise. These tools include Python and R for programming-centric approaches, SAS and IBM SPSS for comprehensive customer data analytics, RapidMiner and KNIME for user-friendly interfaces, and cloud-based platforms like Azure Machine Learning and Google Cloud AI Platform.
Collateral challenges
Predictive customer analytics challenges exist due to the inherent complexity of harnessing data-driven customer insights for business advantage. While predictive analytics offers numerous benefits, such as proactive decision-making and competitive advantages, it demands a robust data infrastructure, expertise in data science, and vigilant monitoring for potential pitfalls like data utilization issues, biases, and model interpretability.
We compiled the most common challenges businesses face when adopting predictive behavior modeling for business and added a few tips to help you address those hurdles.
1. Data quality and availability: incomplete, inaccurate, or insufficient data can undermine the accuracy of predictive models.
Prevention tip: invest in data cleansing and quality assurance processes. Ensure data collection methods are robust, and consider leveraging data augmentation techniques to enhance data completeness.
2. Bias and fairness issues: predictive models can inherit biases from historical data, leading to unfair or discriminatory outcomes.
Prevention tip: regularly audit and assess models for biases. Employ fairness-aware machine learning techniques and carefully curate training data to reduce bias.
3. Model interpretability and explainability: complex predictive models may lack transparency, making it difficult to explain their predictions.
Prevention tip: use interpretable models where possible and explain model outputs clearly. Additionally, consider adopting model-agnostic interpretability tools to enhance transparency in complex models.
The dynamic nature of customer behavior and the continuous evolution of technology introduce challenges in staying current and adapting analytics strategies to changing market dynamics. These challenges underscore the importance of a holistic approach to data management, a commitment to ethical AI practices, and ongoing investments in both technology and talent to fully harness the potential of predictive customer analytics.
Ethical Considerations in Predictive Customer Analytics
By prioritizing user privacy, you lay the foundation for forging more enduring and robust connections with your audience. With advanced analytics, you can leverage aggregated customer behavior insights without relying on the user’s identity. This allows you to base your decisions on comprehensive engagement data while maintaining the privacy of your customers.
The main principles of customer lifecycle management involve transparency, fairness, and ethics. Businesses should be transparent with customers about how their data is used, providing clear privacy policies and obtaining informed consent for data collection. Fairness entails avoiding discriminatory practices and ensuring that predictive models do not perpetuate bias.
Ethical considerations involve using data and predictions for legitimate purposes that benefit both customers and the business while respecting individuals’ privacy rights and adhering to relevant regulations, such as GDPR or CCPA.
Data security and protection should also be prioritized to protect customer information from unauthorized access or breaches. As an experienced analytics services company, Lightpoint can provide your business with tailored solutions for safeguarding your customers’ data and complying with the related regulations.
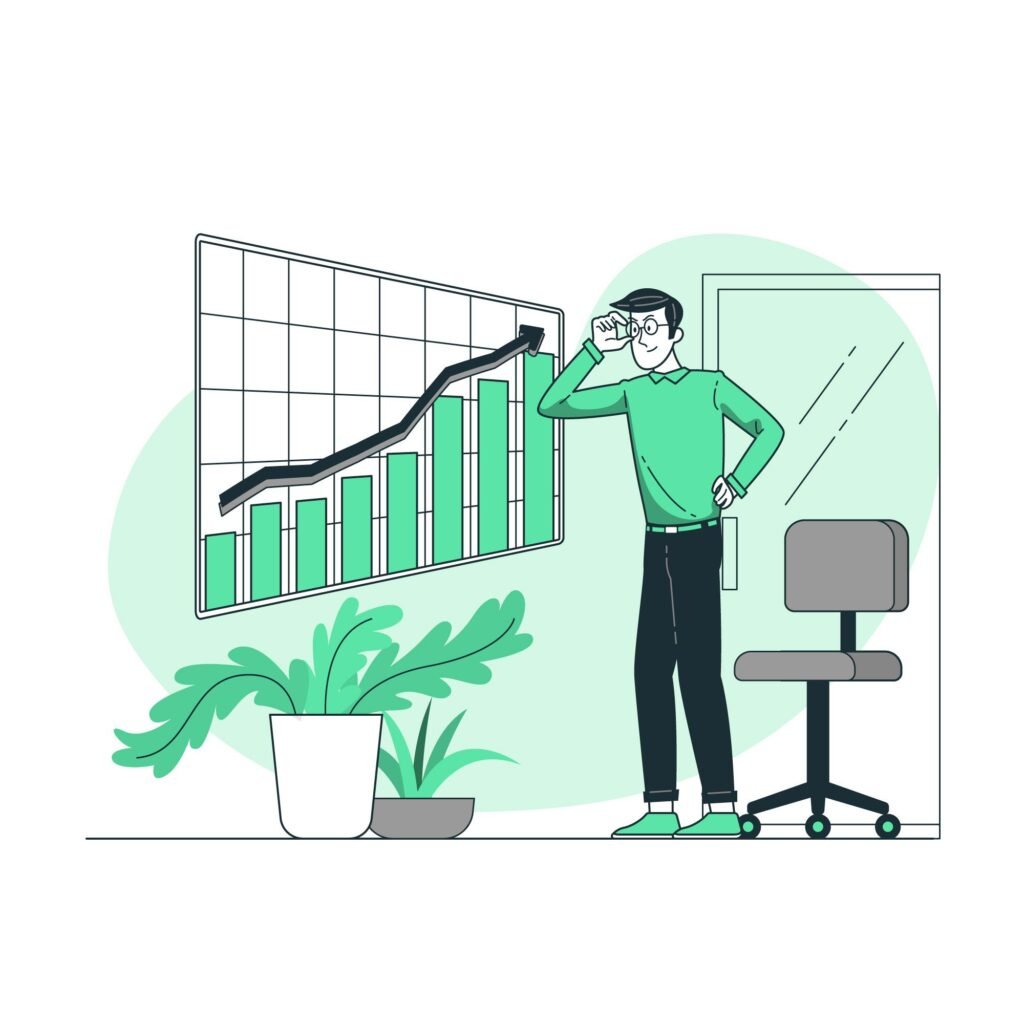
Future Trends: The Evolution of Predictive Customer Analytics
In 2018, the worldwide market for risk analytics was valued at $18,253.49 million, and now it’s estimated to grow to $65,253.66 million by 2026. Risk analytics software helps organizations handle various risks more efficiently. It can identify and manage different types of risks while also taking steps to prevent potential crises. The global market is growing due to increased cyberattacks, fraud, and data theft as businesses embrace digitization trends.
Therefore, any forecasting technologies will keep growing as predictive analytics can directly mitigate collateral risks for businesses.
Predictive customer analytics is expected to evolve in the following ways:
- It will become increasingly sophisticated, leveraging AI and machine learning to offer hyper-personalization. Businesses will deliver highly tailored experiences, product recommendations, and targeted marketing messages, resulting in deeper customer engagement and loyalty.
- Future trends will focus on real-time predictions. Businesses will move from historical insights to instant, data-driven decision-making, enabling immediate responses to customer behavior and market shifts.
- Ethical considerations will gain prominence. Predictive customer analytics will evolve to incorporate fairness and transparency, addressing biases and ensuring responsible AI practices to maintain customer trust and comply with regulations.
- Predictive customer analytics will integrate unstructured data sources like social media, customer reviews, and voice interactions. Natural language processing (NLP) and sentiment analysis will significantly extract insights from these sources, providing a more holistic view of customer sentiment and behavior.
A successful evolutionary concept, forward-looking planning, is particularly necessary in our increasingly complex world. Of course, not just in retail.
The cornerstone of marketing strategies in any industry is customer behavior, habits, desires, and reactions. In this regard, there are some trends that e-commerce businesses can use to train algorithms and create predictive models for recognizing patterns of user behavior in the coming year. In July 2023, McKinsey research highlighted four key trends that can help marketers in consumer behavior forecasting for 2024:
1. E-commerce and physical retail convergence
A blend of online and in-store shopping experiences increasingly marks consumer behavior. Shoppers now expect seamless transitions between digital and physical channels, emphasizing the need for omnichannel strategies that offer convenience and personalization.
2. Sustainability and health-conscious choices
Consumers are becoming more environmentally and health-conscious. They seek products that align with their values, driving demand for sustainable and healthy offerings. Brands that prioritize sustainability and transparency are gaining favor among consumers.
3. Digital engagement and personalization
The digital landscape continues to shape consumer behavior, emphasizing personalized experiences. Consumers expect tailored recommendations and content, making data-driven personalization strategies for businesses to stimulate proactive engagement.
4. Social responsibility and ethical brands
Consumers are increasingly supporting brands that demonstrate social responsibility and ethical practices. Brands that align with social causes and show commitment to ethical production and sourcing are winning consumer trust and loyalty.
These trends highlight the evolving consumer landscape, where digitalization, sustainability, personalization, and ethical values are pivotal in shaping purchasing decisions. Also, they will be a valuable informational asset for businesses to derive actionable insights from predictive customer analytics in 2024.
Data science services make it possible to forecast events, trends, and activities and generate operational or strategic benefits. In the future, online retailers will be able to use predictive models to convert the customer insights generated from predictive analyses into their own competitive advantages.
Conclusion
Predictive algorithms has revolutionized how companies enhance data-driven decision-making as well asgenerate insights and predictions to identify opportunities, minimize risks, and improve operational efficiency.
To turn measurable insights into customer behavior and embrace predictive analytics as a strategic tool, take note of this advice:
- Connect data and data strategies
- Design intelligent automated processes
- Produce creative, relevant content
- Stay agile in your customer focus
Predictive analytics applications are diverse, ranging from marketing and sales forecasting to fraud detection and preventative maintenance. To begin with, schedule a consultation with a Lightpoint expert to identify the most promising starting point.